Covey and Smart Citizens sensor networks
01 February 2017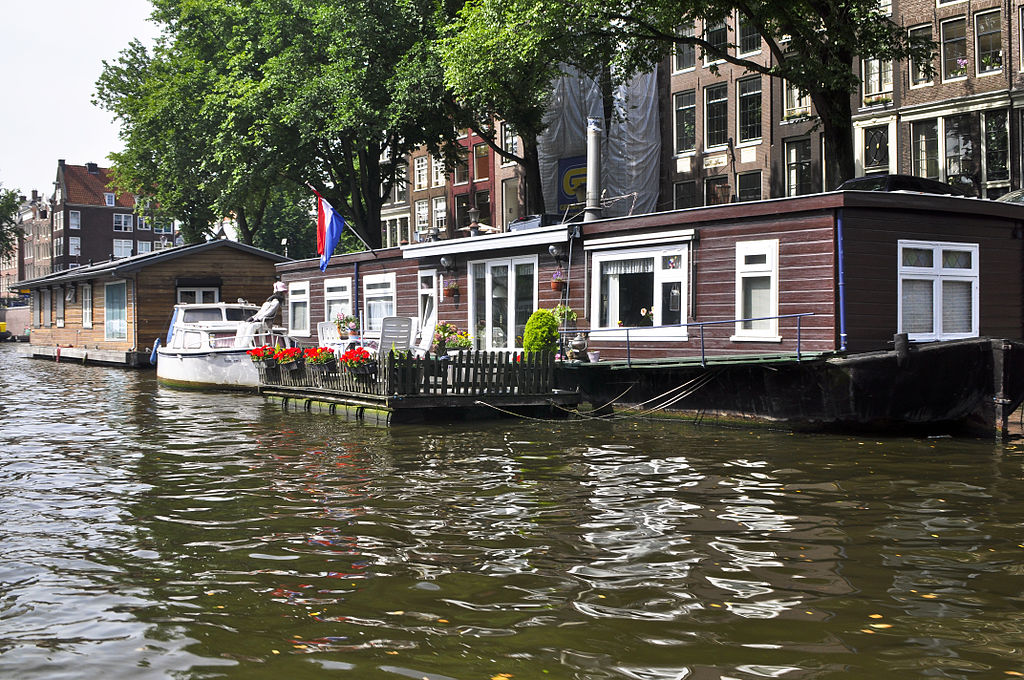
What can we learn from Covey’s Seven Habits when designing sensor networks?
In a recent article I have been stipulating that in order to build successful sensor-networks around a certain environmental issue, we need to combine the elements of (1) concern, awareness) and (2) frustration due to lack of data to start the project. But, in order to keep citizens involved, it might be a good idea if we present people not just with numbers, but (3) compile these numbers into actionable information.
In this follow-up article I want to track back a little to uncover another design-aspect of successful networks, and that is the chosen research-parameter. Often pilots start with a research-parameter that could indeed be measured from a technological point of view, but still fails to pass the third rule since it is not possible to deliver actionable information with the sensor.
Example: Measuring NO2
Imagine that you have come across a brand-new NO2-sensor that you could easily connect to smartphones. As you know, NO2 is a huge environmental issue especially within cities, so this sounds like a great opportunity for a meaningful Citizen Science project. You write a proposal, get funded, compile the code, develop an app and the data-collecting sky-rockets from day one.
You’ve done your homework well, so you deliver each user with direct feedback of his or her own data complete with benchmarks, WHO-guidelines and actionable information. Somewhere in the second month, you are asked to compile some early results: is the air cleaner or dirtier at the Dam central square in Amsterdam than can be derived from calculated models?
Great, enough data about the Dam with all the tourists and locals around! Enthusiastically you start analyzing, but alas .. pretty soon you run into some minor trouble. Yes, NO2 is everywhere, and levels vary wildly from place to place and time to time. And yes, measurements show that the NO2 levels can be way beyond WHO-guidelines. But when you take a second look at the data, you will often find that what looked so promising and useful at first sight, cannot be compiled into usable reports. How come?
Smartphones tend to be stored inside coats and jackets at least part of the time, not a perfect environment to sniff the flow of air. Of course they do get a lot of outside air, but how can we determine what was measured at 12:55:34 with Smartphone #34287? That sudden rise, is that a bus passing by? Or does the owner steps into a crowded tramline? Or walks by a Dutch coffeeshop? When looking at the location data, it will often be impossible to tell if it reflects inside or outside conditions. Since the claim was to measure NO2 in the outside environment, you have to somehow filter on that. The lack of handles to do so, however, causes the project to loose steam and you’re left wondering what went wrong.
Covey steps in.
If we want to determine the main cause of the demonstrated problem, we have to return to the beginning of this story: a new sensor was available that could be connected easily to a Smartphone. But the fact that this is technologically feasible, does not guarantee a successful measuring network. Technology-pushed solutions are introduced actually quite often and can lure even the biggest of organizations into dead alleys. How can we prevent this from happening?
Basically there are two things to consider before you start, and the most important is to stick to Covey’s most important theorem and begin with the end in mind. If you want to measure NO2 to be able to say something about the air quality along a certain road, it might be better to plan your sensors alongside that road and not cast a wider net over a whole area, hoping to be able to answer many questions at once, to prevent that in the end you can answer none at all.
The second one is the realization that although many environmental parameters could in principal be measured, not all of them are a good match for a citizen sensor network. Is the parameter you’re looking at highly local? Does it matter if the device is inside a building or car, or outside? Then a fixed, controlled solution could be a much better choice. If the parameter is not highly dependent on a location (but more on an area) and inside and outside measurement can be combined without problems, then mobile measurements become a possibility. A situation that favors multiple types of devices is if there are very clear thresholds for what is unacceptable, independently of where this condition is met. So although it is tempting to look at all sensors as theoretically suitable for a sensor network, it might be wise to reconsider this before you start.
An example: monitoring waves
Let’s look at an example to make this less abstract. Recently ,we spoke at Waag Society about the possibility to monitor wave-movements on houseboats rocking in the Amsterdam canals. Sometimes fast and/or large boats pass by, creating waves that might be experienced on the houseboats as uncomfortable. Could we measure this discomfort with a citizen sensor network?
The sensor itself is easy, as all smartphones can measure movement. So if we could develop an app that owners could install on old, plugged in smartphones, the network itself could quickly and cheaply be realized. There is no standard parameter for this, so we invent a movement indicator we call ‘MI’. Considering privacy-limitations, we want to send in data only above a certain threshold.
This sounds easy, but when trying to working out this threshold, it becomes clear that there are all kinds of house boats: some weigh a few tons, others many times more. So the threshold on boat 1 makes us completely blind for movements on boat 2. Setting individual thresholds based on the mass of participating boats seems risky business as well, since mass is just one variable here. Others variables could be the position on the canal, the length of the boat in meters or the shape of the hull.
So quickly we run literally into a swamp of potential interpretation-troubles, making the project look a whole lot less interesting. But wait, what was the question we had again before we started on the implementation of the technical side of things? You cannot measure discomfort, but maybe we can measure speeds of passing boats or maybe water displacement?
To monitor individual discomfort, focus on the group
If we want to monitor the speed of boats passing by individual houseboats, we need to combine data from multiple sources so that means we can only analyze data cluster-wise. By organizing community meetings we generate enough participation to have at least three or four data-streams per canal that we want to gather data on.
To be able to interpret the data afterwards in the right way, it might be a good idea to rent one or two boats, and drive test-runs past the houseboats on a certain set of fixed speeds. This tells us the distance in meters between the houseboats, and provides us a baseline which we can use to calculate speeds and other things with later on.
As an insurance, we could add a (very low-res) video-stream shot from one houseboat per canal. The different patterns can be categorized and then looked back up in the video stream to be able to label the categories in a meaningful way Now we’ve got all positions covered and we can start the adventure!
To conclude we can state that the project found traction again when we stopped focusing on individual results, and zoomed out again towards the bigger picture. So we do not want to know anything particular about individual houseboats, but use them as a platform to measure the speed of passing boats. This releases us from individual calibration-issues and paves the way for a smooth interpretation of the recorded patterns. The insurance we added by way of the captured video stream, might help us to interpret the data if we would run into interpretation troubles later.
René Post, Waag Society